Exploring the 5 Main Challenges in Data Integration
- Shiva Jannesari
- Jun 19, 2023
- 2 min read
Data integration is a crucial process that allows organizations to extract valuable insights from diverse data sources. However, it is not without its challenges. In this blog post, we will dive into the 5 main challenges encountered during data integration. By understanding and addressing these hurdles, you can optimize your data integration efforts and drive informed decision-making. Let's explore these challenges in detail and uncover strategies to overcome them.

Inconsistent Data Structures: The Integration Puzzle
Data integration often involves combining data from different systems with varying structures. Diverse table layouts, column names, and data organization can pose significant challenges. To overcome this hurdle, it is important to analyze the data structures of source systems and establish a unified schema for integration. Utilizing data transformation techniques and mapping tools can help harmonize and align the structures, facilitating seamless integration.
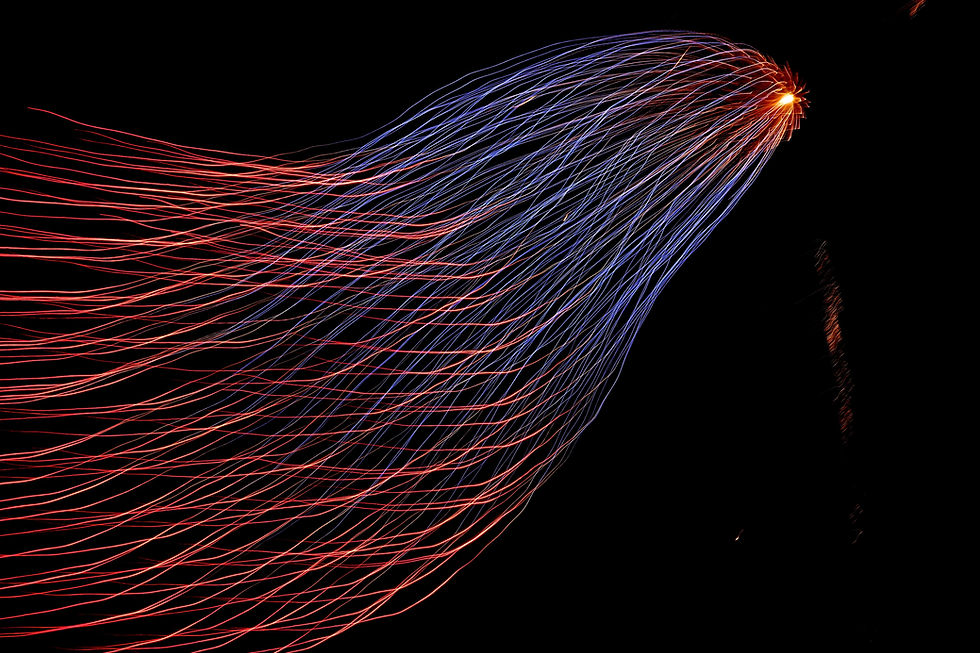
Data Type Mapping and Conversion: Making Sense of Differences
Integrating data from multiple sources often means dealing with different data types. Numeric representations, date formats, and textual data can vary across systems. Mapping and converting data types become essential for consistency and accurate interpretation. By employing robust data type mapping and conversion strategies, you can ensure compatibility between source and target systems, minimizing data loss and ensuring data integrity.
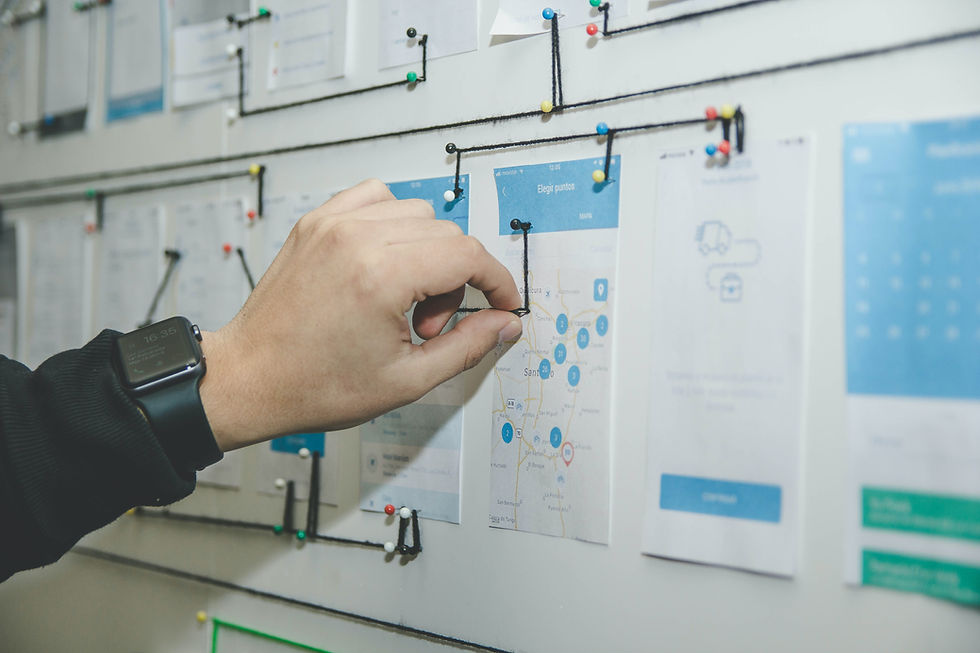
Encoding and Character Set Differences: Preserving Meaningful Data
Data integration across systems with different character encodings and character sets can introduce complexities. Incompatible encodings may lead to data corruption or misinterpretation, especially when handling special characters or non-standard character sets. Normalizing character encodings and employing appropriate conversion techniques are crucial for preserving data integrity and ensuring consistent interpretation.
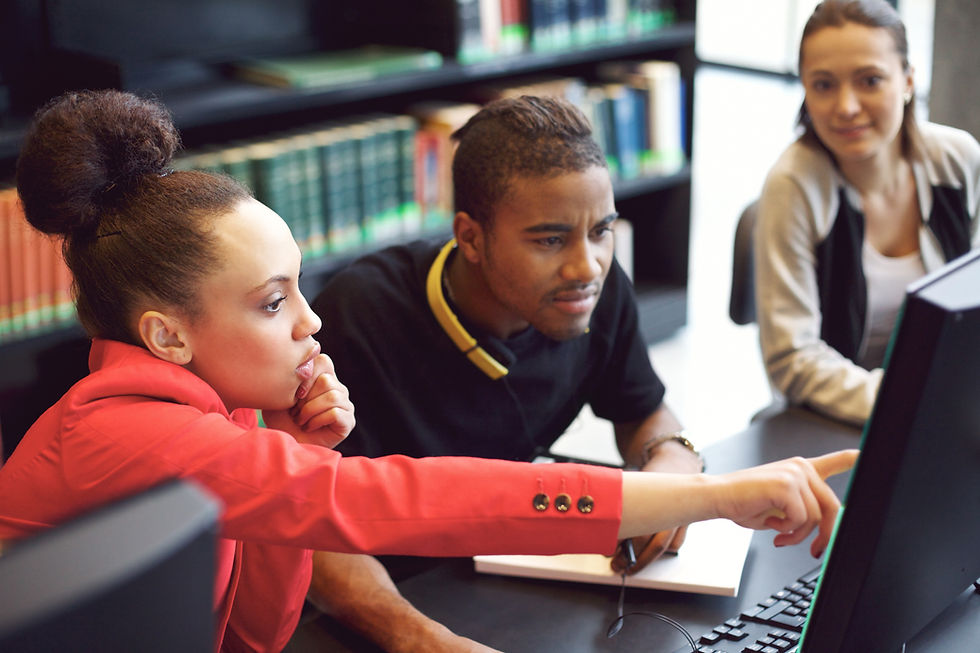
Data Quality: The Cornerstone of Integration Success
Maintaining data quality is paramount for successful data integration. Disparate data sources often contain inconsistencies, missing values, and duplicate records. Addressing data quality challenges involves data profiling, cleansing, standardization, and deduplication. By implementing a robust data quality framework, you can enhance the accuracy and reliability of integrated data, enabling confident decision-making.

Bridging the Semantic Gap: Aligning Meaning and Context
Integrating data from diverse sources requires addressing semantic and contextual variations. Different terminologies, units of measure, and business rules can hinder seamless integration. Bridging the semantic gap involves establishing a common vocabulary, mapping data elements to a standardized ontology, and ensuring clear rules for data interpretation. By aligning context and meaning, you can ensure consistent understanding and enable accurate analysis across integrated datasets.

Data integration brings immense value to organizations, but it comes with its share of challenges. By recognizing and addressing the 5 main challenges of inconsistent data structures, data type mapping, encoding differences, data quality concerns, and semantic variations, you can navigate the integration process successfully. Embrace these challenges as opportunities for improvement and leverage effective strategies and tools to overcome them. With a well-executed data integration strategy, you can unlock the true potential of your data assets and make data-driven decisions that drive your organization forward.